The term “quality control” became an essential part of manufacturing since Henry Ford’s first assembly line debuted in 1913 (and perhaps even before that). By creating uniform parts and assembling them in a specific, repeatable manner to produce identical results, goods can be mass produced at lower cost.
Quality control helps to minimize the risk of product recalls, customer complaints, and lost revenue. It enhances a company's reputation by demonstrating a commitment to producing high-quality products that meet customer expectations. As a member of the manufacturing community, you probably know all this. What you might not be familiar with yet are the ways in which Industry 4.0 technologies have vastly improved how quality can be managed.
To understand the progression, we’ll explore three broad stages that quality control has gone through:
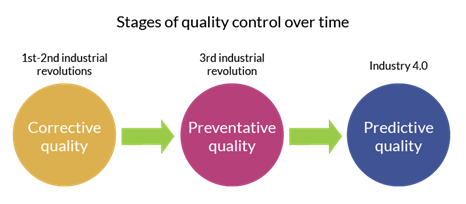
Let’s briefly walk through how each stage developed over time, to better understand the historical context of Industry 4.0’s new approach to quality.
Corrective quality control
When mass manufacturing began, quality was controlled through corrective measures. Quality inspectors worked on the manufacturing line, measuring and inspecting parts by hand and using simple tools.
Defective parts were identified after production was complete and reworked to conform to quality specifications. All parts that could not be reworked would be scrapped. This approach was quite inefficient, since rework took quite a bit of time and valuable manufacturing time was spent on some parts that wound up in the scrap pile.
With corrective measures alone, manufacturers found it challenging to prevent quality issues: there was little consistency in how the process was controlled and even less insight into what caused the defects. We now know they collected insufficient data to track patterns and changes on the line.
Preventative quality control
As manufacturing technology evolved, manufacturers started to take a more scientific approach to quality and introduced preventative quality methodologies such as Statistical Process Control (SPC) and automated in-process testing.
Using SPC and automated in-process testing, machines could monitor parts to ensure they fell within tightly defined specification limits at various stages in the manufacturing process. These processes provided new insights and it became possible to see when certain machines or stations were creating bad parts with greater accuracy. Process engineers could determine which points in the process were breaking down. Parts were still often reworked and scrapped, but at least there was an opportunity to analyze manufacturing data and find root causes – although getting to these conclusions required many hours of manual analysis by teams of data scientists.
The downside to using these preventative methods are that when they are used alone, the information they provide is often too little, too late. Many bad parts could be created before the manual methods of SPC analysis can trace where the problem originated. The sheer volume of manufacturing data makes it challenging to work with.
The Industry 4.0 approach: predictive quality
Industry 4.0 has ushered in a wave of digitization to manufacturing, through the Industrial Internet of Things (IIoT) and technologies such as autonomous robots, additive manufacturing, artificial intelligence, and machine learning.
AI and machine learning are particularly adept at processing and analyzing vast amounts of big data with high precision. For example, they are perfectly suited to assisting humans with the analysis of manufacturing data that automotive precision manufacturers now collect during production. Artificial intelligence and machine learning have made a new method of quality control available in manufacturing: predictive quality control.

Predictive quality software solutions – like Acerta’s LinePulse – offer more traditional SPC analysis and also use machine learning and AI to enable advanced analytics and the ability to process data at lightning speeds. This type of software monitors data signals and applies them against historical patterns, creating alerts in real time if it detects suspicious patterns.
By “learning” how signals should behave, the predictive quality software can identify a potential problem long before a signal would have exceeded its control limits. The predictive quality solution can predict when quality issues will occur so line operators and engineers can intervene sooner to prevent them.
By using machine learning and AI, a predictive quality software solution can also analyze multiple data signals simultaneously and find relationships between them. This offers opportunities to discover problems on the line that would have previously gone undetected.
How does this work? Here’s an example:
In the engine assembly process, a block and cylinder head are bolted together on the assembly line. Let’s imagine that before they are bolted, a cutting machine produces a less-than-flat surface on the block due to some wear on the blade, but the vibration signal of the cutting tool stays within specification limits. When the pieces are bolted together later, some variations are found in torque, speed, and angle at this station. Some of these engines are finished with a tiny gap between the surfaces, but the gap was not detected.

When signals from the cutting tool and the torque tool are analyzed together, however, a pattern emerges and the issue can be traced back to the worn cutting blade. This is how predictive quality software offers a new layer to solve complex manufacturing problems.
Benefits of predictive quality over alternative quality methods
Real-time insights into production mean that a manufacturer can deal with quality issues proactively. The result is that scrap and rework are reduced and overall quality increases. Additionally, some time-consuming and costly quality control processes such as end-of-line testing can be minimized or avoided. (This e-Book explains how predictive quality software can reduce a manufacturer’s reliance on end-of-line testing.)
Predictive quality software empowers front-line production staff with advanced analytics that were previously only accessible to data scientists. When all quality data from multiple manufacturing lines can be accessed in a single dashboard, it makes problem-solving faster and easier.
An overall increase in quality and efficiency during production can maximize productivity for manufacturers. And, addressing quality problems proactively during the manufacturing process means that fewer defective parts will be produced, reducing downstream quality spills and ensuring the manufacturer stays competitive in today’s market.
Sign up today for a free Essential Membership to Automation Alley to keep your finger on the pulse of digital transformation in Michigan and beyond.